How a Cloud-Based Big Data Solution Can Benefit Your Business
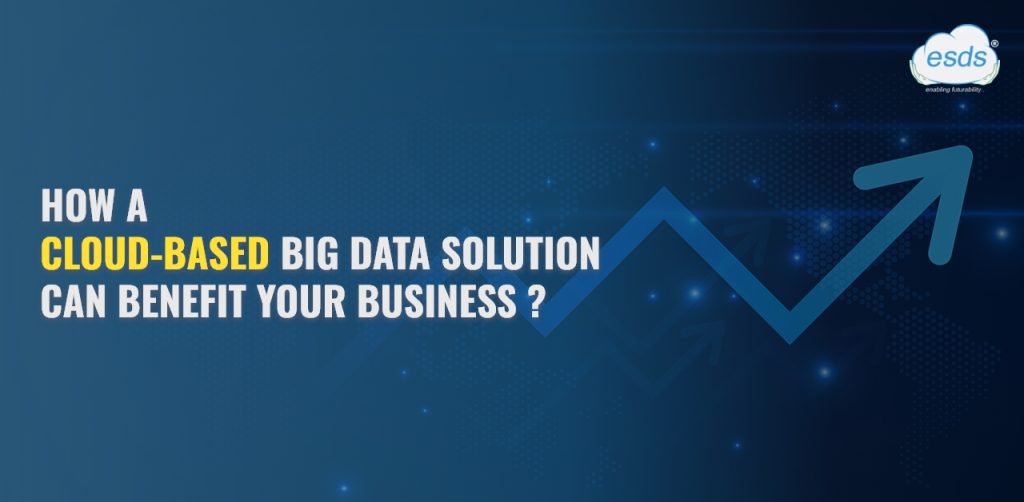
Big data has become essential to corporate operations in all industries in today’s fast-paced digital world. Organizations looking to use this priceless resource face opportunities and problems due to the sheer volume, diversity, and velocity of data created daily. Cloud-based big data solutions have become a game-changer amidst this data flood, providing unmatched scalability, flexibility, and cost-efficiency. A McKinsey analysis claims that cloud-based big data solutions may boost EBITDA by 7–15%, reduce IT costs by 10%–20%, and accelerate product development by up to 30%. According to a different McKinsey & Company research, businesses that successfully employ big data and analytics have up to a 23-fold increase in client acquisition, a 9-fold increase in customer retention, and a 26-fold increase in profitability compared to their competitors.
Businesses must do several things right for their cloud shift to be successful, starting with choosing the optimal cloud deployment architecture. Let’s briefly review the alternatives that are accessible.
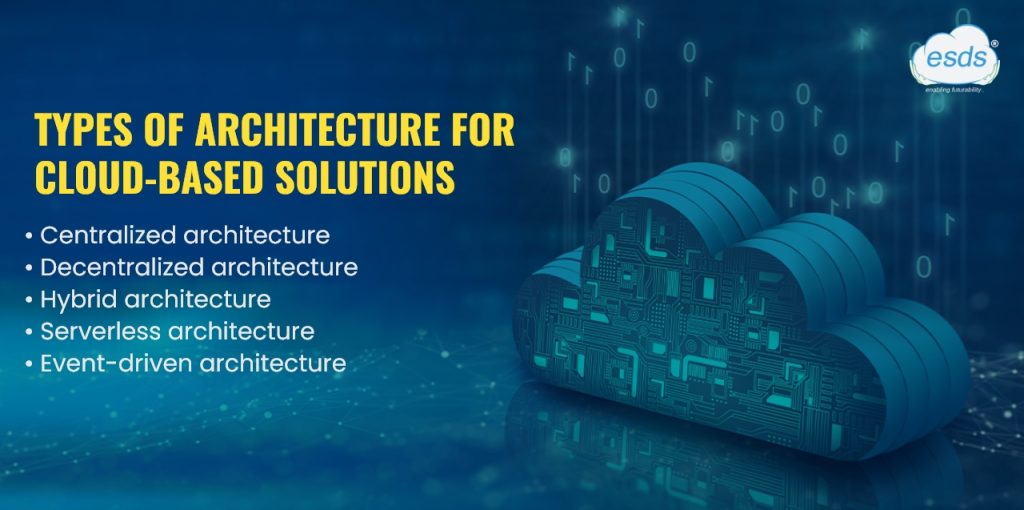
Types of Architecture for Cloud-Based Data Solutions
Below are the types of cloud deployment for big data platforms you can choose from the following: –
- Centralized architecture
- Decentralized architecture
- Hybrid architecture
- Serverless architecture
- Event-driven architecture
The cloud architecture you choose will rely on your business requirements. These are each type’s primary characteristics.
- Centralized architecture: –
Centralized architecture is the way of storing the incoming data, which is collected in a single storage account known as the data warehouse. This combines data from different domains like financial data, social media, payroll numbers, and other distinct subjects. This helps you to create a better comprehensive picture of your business and ensures that the data is owned by the central IT department.
While this requires a lot of data processing and storage resources, it is easier to analyze. However, you can consider the decentralized architecture as an alternative option.
- Decentralized architecture: –
This method avoids copying data from different subjects/domains into a single data lake/warehouse. Rather, it is kept in several data lakes that are accessible from a single storage account. This speeds up time-to-value, permits dispersed ownership of the data, and minimizes the ETL data pipeline.
However, there are several trade-offs:
Lack of data versioning because there is no single data perspective results in a slower system than one that is centralized; disruptions at data sources disturb the chain of operations
For this reason, you can choose to use a hybrid big data architecture, which combines the two aforementioned kinds.
- Hybrid architecture: –
In this strategy, structured data is combined with a federated data lake and unstructured data with a centralized data warehouse. Hybrid data lakes can be used as inputs for data science or machine learning projects. If not, this data may be transformed into structured data in a data warehouse and utilized for analytics and business intelligence using a typical ETL pipeline.
All these methods, however, demand massive resources to be ready, and data sources may not consistently generate data at consistent speeds or quantities. This implies that these resources may stay inactive for an extended period, which is not ideal. Serverless architecture is required due to resource conservation considerations.
- Serverless Big Data Architecture: –
Serverless big data architecture refers to a setup processing and analysis of large amounts of data that utilizes serverless computing technologies to handle large amounts of data without the need for managing servers or infrastructure. In this big data architecture, data processing tasks, such as data ingestion, transformation, analysis, and storage, are executed in a serverless environment where the cloud service provider dynamically manages the allocation and scaling of resources based on demand.
- Event-driven architecture: –
Event-driven architecture for big data involves leveraging the principles of event-driven design in the context of processing and analyzing a large amount of big data. It enables time processing of data streams generated by various sources such as sensors, applications, social media feeds, and IoT devices.
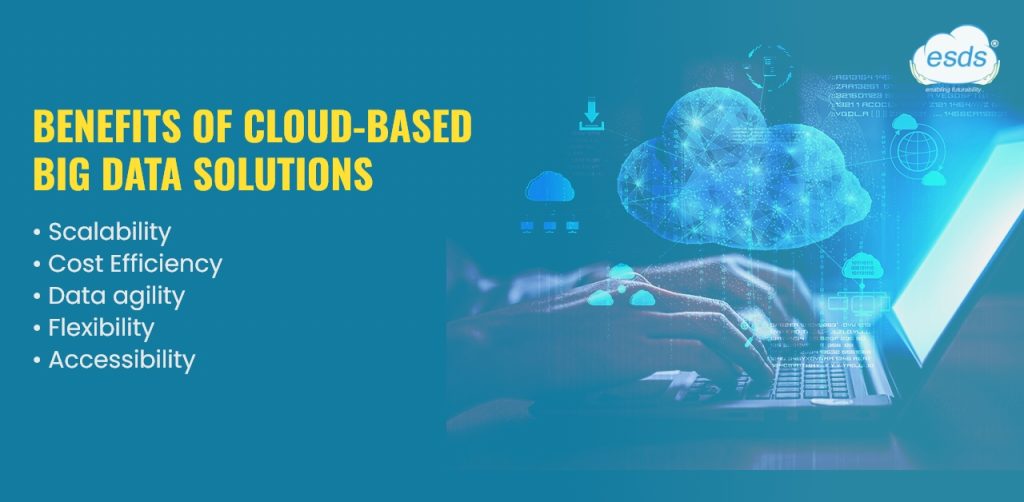
Benefits of Cloud-Based Big Data Solutions
Cloud-based big data offers multiple benefits, revolutionizing the way organizations handle and derive insights from vast amounts of data. Below are some of the key advantages:
- Scalability: –
If you anticipate frequent changes in the needs for your data input pipeline, you may plan the spinning up or down of cloud instances whether your big data solutions are hosted in a public, private, or hybrid cloud. As an alternative, you may configure your big data architecture to scale up or down automatically based on the number of resources being used. This guarantees that your analytical solution can manage any data quantities that your company objectives may call for.
- Cost efficiency: –
With accurate resource allocation, data burden balancing, and failover scenarios, you won’t ever squander cash on unnecessary cloud resources. A lot of automation, defined workload thresholds, and automatic triggers like webhooks, API requests, and other technologies are needed to scale up and down on demand. To avoid overpaying for idle instances, this may guarantee that your infrastructure is economical and shuts down as soon as the demand spike passes.
- Data agility: –
To obtain valuable insights from big data, it is essential to analyze incoming raw data as fast as feasible. Because of its agility, your big data platform will be able to manage any workload regardless of the underlying data architecture, swiftly, reliably, and at the required scale.
- Flexibility: –
Big data solutions are adaptable because they may expand or contract to satisfy updated sets of analytical requirements. Data flexibility also indicates that the analytics outcomes are affected by the way the data is converted before processing, in addition to the circumstances and factors of data input. Data sources are always changing; therefore, data analytics solutions need to be adaptable.
- Accessibility: –
Although big data may be ingested from many different sources, it will still be unsorted, appear in unclear formats, have incorrect headers and column types, have erratic encoding, and more. To make the data more easily analyzed and hence more valuable, it must be arranged, standardized, cleaned of duplicates, converted, and packed into a visible schema. The use of cloud deployment guarantees that you will always have enough resources to carry out analytics in real time.
Challenges of Big Data Solutions
Ensuring big data security and compliance, creating transparent data management, and expediting tool integration are the three main problems of big data processing.
- Data security and compliance concerns: –
Robust security measures are necessary for cloud-based big data analytics solutions that have several data input points. Strict security standards must be followed while collecting, accessing, processing, and storing personal data, such as postings made on social media and other PII (personally identifiable information). HIPAA, for instance, has regulations for the management of research findings, medical imaging, and electronic health records (EHRs).
Make the most of the security capabilities offered by your cloud platform and, if necessary, take further precautions. In certain situations, cloud computing might transfer risk from you to the service provider; nevertheless, you are still in charge of choosing the right data formats and managing them safely.
- Challenges in data management: –
Your big data solution might gather unstructured data, often incomplete and impossible to validate. Therefore, incorporate mechanisms for data validation and correction (e.g., updating databases, repairing data sources, and using reliable data gathering agent authentication methods). The output of data validation procedures is structured data ready for use in business intelligence tools, like analytics and training machine learning models.
Conclusion
Running big data analytics is critical for attaining these aims. However, it presents various obstacles, ranging from data security and dynamic infrastructure expansion to interoperability with third-party applications. Cloud implementation improves cost-effectiveness, scalability, data processing quickness, flexibility, and operational reliability. To ensure flawless implementation and gain these benefits, you should plan, develop, and execute a cloud migration utilizing industry best practices.
ESDS has eNlight’s Cloud Platform that offers a fully managed Big Data framework, making it quick, simple, and cost-effective to handle massive volumes of data over a dynamically scaled eNlight cloud. Big Data as a Service safely and securely manages a wide range of big data use cases, including log analysis, data transformations (ETL), web indexing, financial analysis, machine learning, scientific simulation, and bioinformatics.