How ML & Predictive Analysis is Revolutionizing Network Security
Network security has become increasingly important in recent years as businesses and organizations rely more heavily on technology to store and process sensitive data. However, with the rise of sophisticated cyber-attacks and the ever-increasing complexity of networks, traditional security measures such as firewalls and antivirus software are no longer sufficient to keep networks safe.
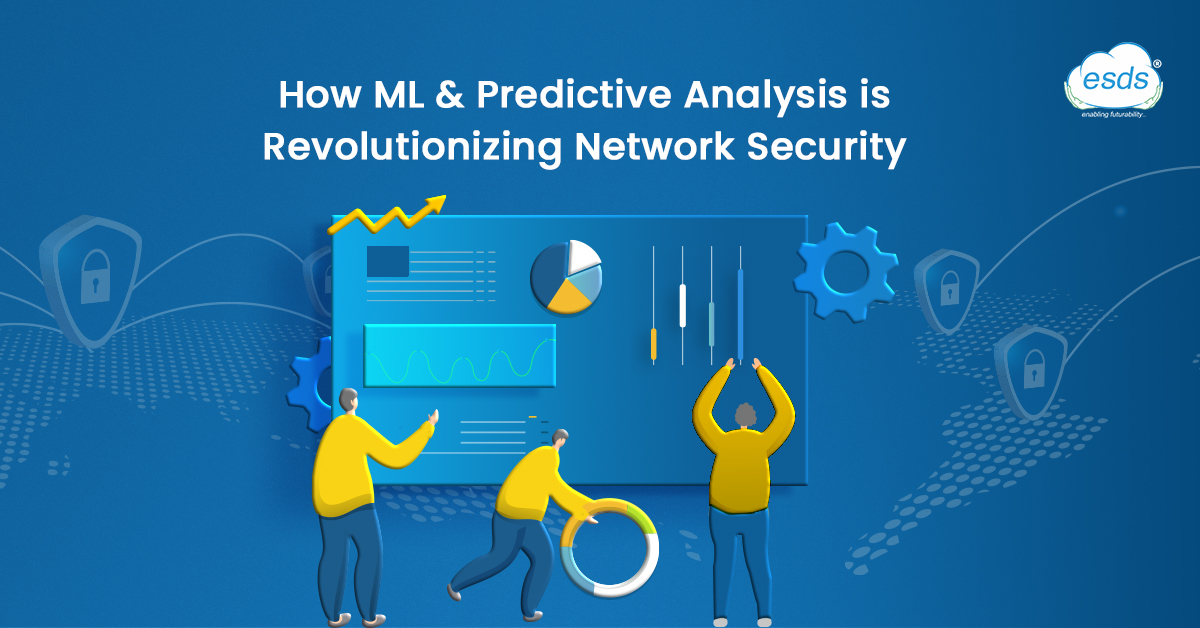
To address this challenge, predictive analytics and machine learning (ML) have emerged as powerful tools for network security. By analyzing large amounts of data and identifying patterns and anomalies, these technologies can help identify and respond to threats more quickly and effectively than traditional security solutions.
The Role of Predictive Analytics and ML Network Security Solutions
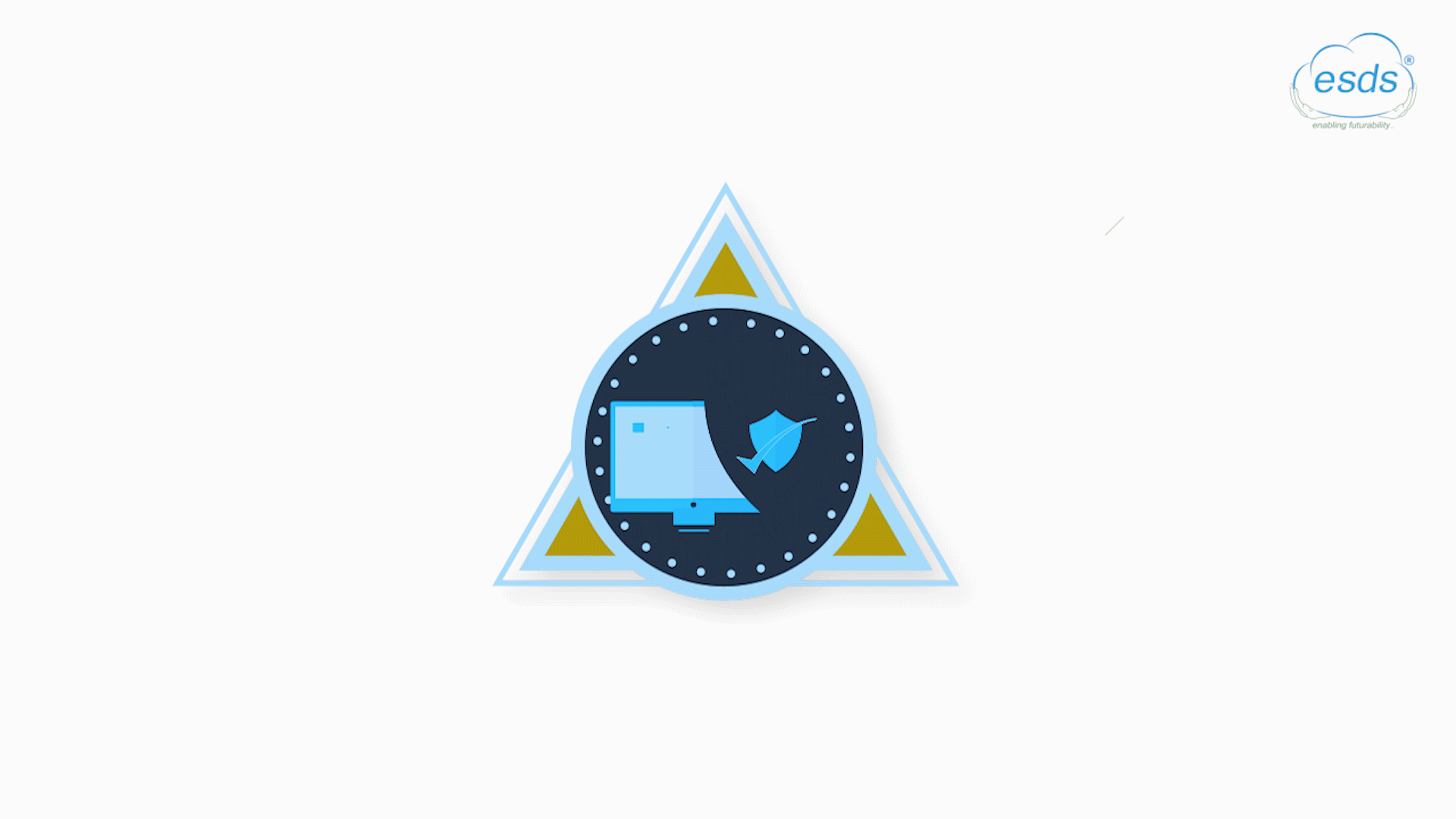
The term “ML-driven network security solutions” in cybersecurity refers to automating various threat detection processes through self-learning algorithms and other predictive technologies (statistics, time analysis, correlations, etc.). Due to the limits of conventional rule-based security solutions, the usage of ML algorithms is growing in popularity for scalable technologies. As a result, data is processed using sophisticated algorithms to spot trends, abnormalities, and other subtle signs of malicious activity, including emerging threats that might not yet have established signatures or known bad indicators.
A key component of general cyber hygiene continues to be identifying recognized threat indicators and blocking known attack methods. Nevertheless, when it comes to maintaining and covering all the various log sources, typical systems like threat feeds and static rules can take a lot of time. In addition, the availability of Indicators of Attack (IoA) or Indicators of Compromise (IoC) at the moment of an attack may also be compromised or become quickly outdated. As a result, businesses need alternative strategies to close this hole in their cybersecurity posture.
How predictive analytics & ML can improve network security?
One key advantage of predictive analytics and ML is their ability to learn from past attacks and quickly adapt to new threats. As new threats emerge, these technologies can quickly identify and respond to them without human intervention.
Another advantage of these technologies is their ability to identify and respond to low-level threats that may take time to become apparent to human operators. Predictive analytics and ML can identify patterns and anomalies that may indicate a potential threat by analyzing vast amounts of data from network logs, intrusion detection systems, and other sources.
Benefits of Predictive Analytics & ML for Network Security
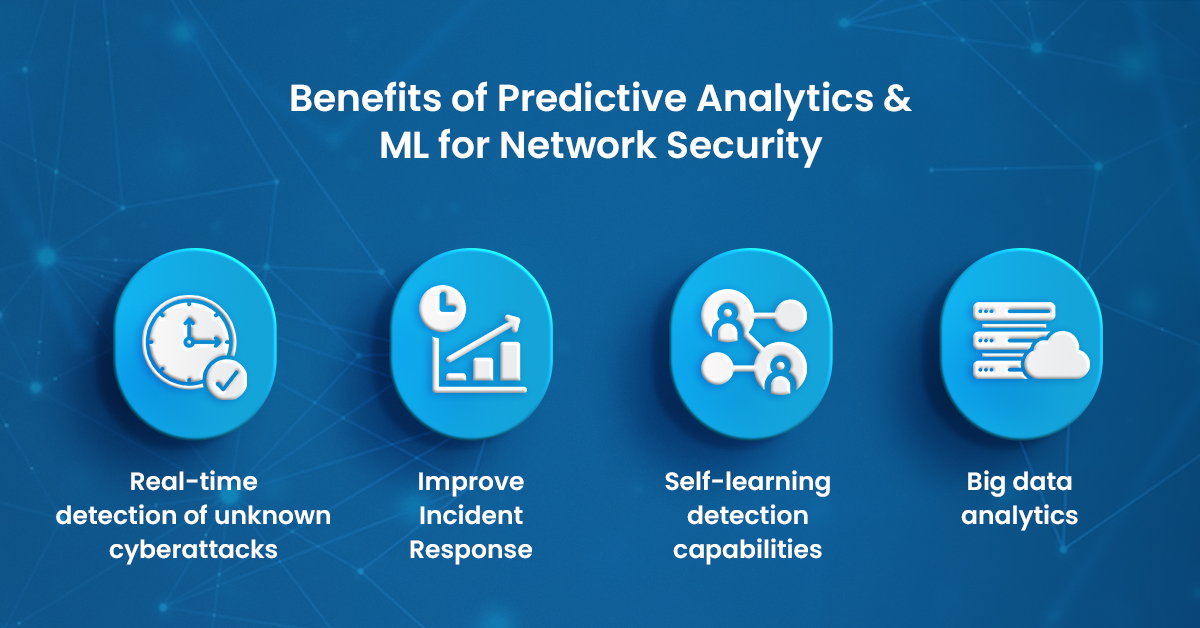
Real-time detection of unknown cyberattacks: While it is straightforward to immediately identify known malicious indicators (specific IP addresses, domains, etc.), many attacks may go undetected when these indicators are absent. If so, statistical, timing, and correlation-based detections are extremely valuable for automatically identifying unknown attack patterns. In addition, by using algorithmic approaches, traditional security systems based on signatures and indicators of compromise (IoC) can be improved to become more self-sufficient and less dependent on known malware indicators.
Improve Incident Response: ML can automate some portions of the incident response process, reducing the time and resources needed to deal with a security breach by learning from an analyst’s prior incident response efforts. This may entail using algorithms to text and evidence analysis to pinpoint attack patterns and core causes.
Self-learning detection capabilities: ML-driven solutions improve their threat detection, threat scoring, clustering, and network visualizations continually by learning from previous occurrences. This could entail training the algorithms directly or changing how information is displayed based on analyst response.
Big data analytics: Organizations must be able to process enormous amounts of information in real-time, including network traffic logs, endpoints, and other sources of information about cyber risks, due to the ever-increasing amount of data and various log sources. By seeing trends and anomalies that could otherwise go unnoticed, ML algorithms might help in this area to detect security threats. Thus, a crucial requirement for threat detection capabilities should be a solution’s capacity and flexibility to integrate various log sources.
There are several areas where predictive analytics and ML can be beneficial for network security.
These technologies can be used by IT Security teams to:
- Identify and respond to advanced persistent threats (APTs), which are long-term, targeted attacks that aim to steal sensitive data or disrupt network operations.
- Monitor network traffic for suspicious activity, such as unusual data transfers or attempts to access sensitive areas of the network.
- Identify potential insider threats, such as employees attempting to steal data or sabotage network operations.
- Analyze user behavior to identify patterns that may indicate a potential threat, such as users who are logging in from unusual locations or accessing sensitive data outside of their regular work hours.
Machine Learning Applications in Network Protection
- Cyber Threat Identification
- AI-based Antivirus Software
- User Behavior Modeling
- Combating AI Threats
- Email Monitoring
Predictive analytics and ML-driven solutions require large amounts of high-quality data to be effective. Therefore, organizations must invest in data collection and analysis tools like VTMScan and hire skilled data analysts and security professionals or have managed SOC services to interpret the data and make informed decisions.
Overall, predictive analytics and machine learning will likely drive the future of network security. As cyber-attacks become more sophisticated and networks become more complex, these technologies will play an increasingly important role in keeping networks safe and secure.
Ready to put what you’ve learned into practice? Get the best Security Solutions
- Decoding Generative AI: A Comprehensive Guide to Gartner’s Impact Radar - January 2, 2024
- 5 Best Practices for Cloud Security in 2024 - December 29, 2023
- 10 Best Machine Learning Ops Strategies for Cloud Environments in 2024 - December 29, 2023